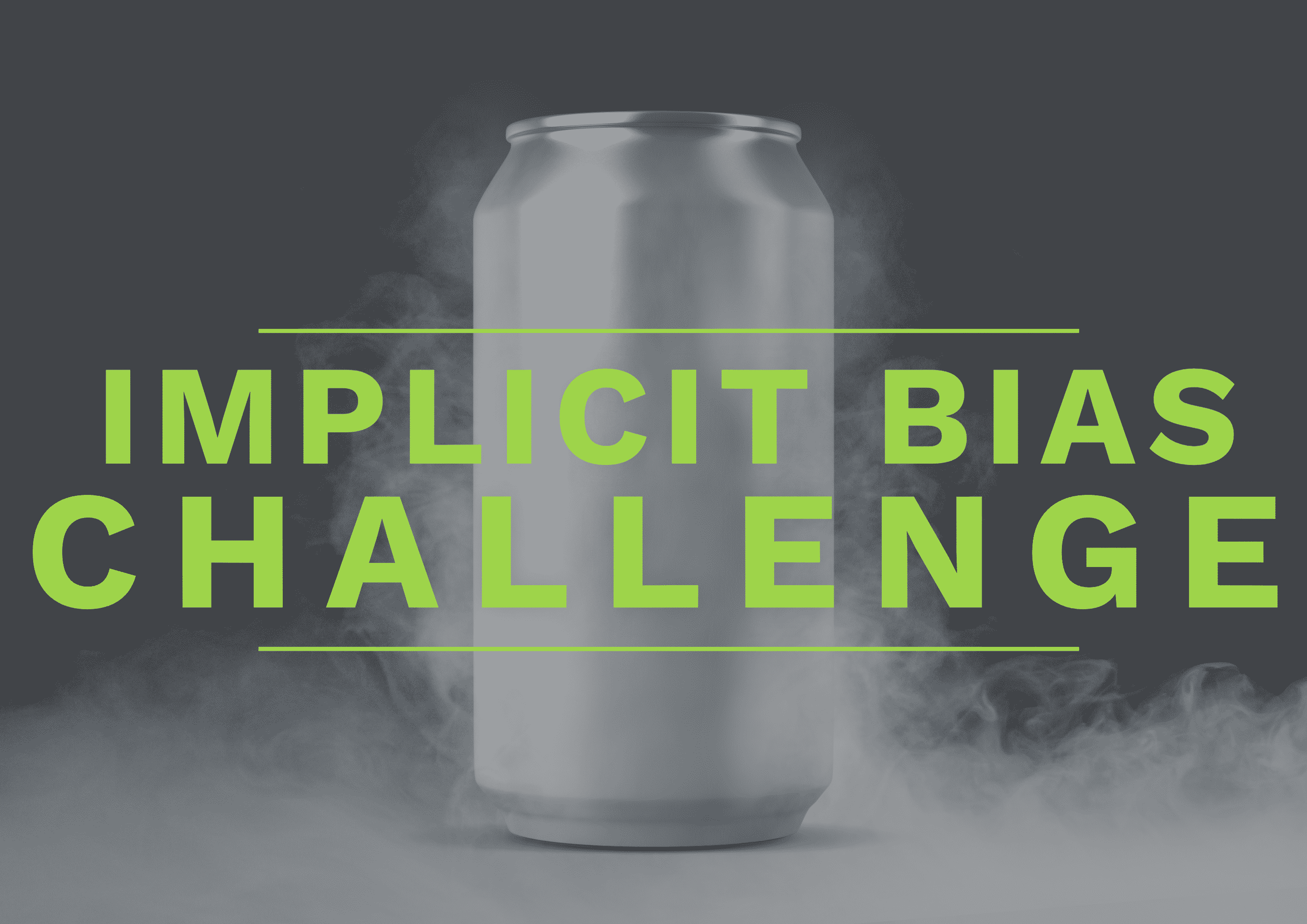
If you were a kid growing up in the ‘80s during the height of the cola wars, chances are you remember the Pepsi challenge. For those of you who may not be familiar, Pepsi went out and set up tables in public areas, covered the soda cans and asked people to pick which one they preferred based on taste. Once the label bias was removed, people regularly chose Pepsi based on taste alone. The airways were filled with Coke drinkers, shocked they preferred Pepsi. This is a classic example of implicit bias.
What’s the key learning?
Once the key identifying data is removed, the bias is also removed.
One of the hottest topics in Talent Acquisition is AI and the implications for streamlining hiring and the potential for removing bias in hiring. But like Amazon learned AI has the potential to automate and scale bias. However, according to Brian Uzzi, professor at The Kellogg School of Management at Northwestern University, “We can simply deny the algorithm the information suspected of biasing the outcome, just as they did in the Pepsi Challenge, to ensure that it makes predictions blind to that variable.”
Is it really that simple?
Not quite, says Dr. Bobbe Baggio, Associate Provost Graduate Cedar Crest College, and Nov Omana, CEO/Founder Collective HR Solutions, “AI, if not carefully programmed and monitored, has the ability to exasperate inequalities in the workplace, home, legal and judicial systems. Sexism, racism, and other unrecognized biases can be built into machine-learning algorithms underlying the intelligence and shape the way people are categorized and addressed. This risks perpetuating an already vicious cycle of bias…The truth is that most of the programming and data analytics are being created globally by white males…has shown that women are less likely than men to be shown ads on Google for executive jobs…these algorithmic flaws are not easy to detect. Ingrained bias could easily be passed on to machine-learning systems and be built into the future.” Removing bias is more than just removing the data; it’s also ensuring that those who are responsible for “setting” the data table are free from implicit bias.
No peaking!
To bring it all back to our cola wars example. If some of the labels showed or if the people were told ahead of time which can was which the label bias would have perpetuated.
We all have label bias.
And, we all have the ability to create a flawed system. So what can we do? The first step starts with each of us. Have you ever measured your own implicit bias? You can test yourself for implicit bias. Take a test here. Why is this important? Each of us, in our own ways, has “tables that we set” every day, and we need to be sure that we are not setting that table with our own implicit bias. For those of us in TA we have an enormous responsibility to constantly check and re-check our own penchant for implicit bias to be sure we are giving everyone a seat at the table and also equal access to those seats.